Genetic Determinism: What it is and how we can avoid it with multi-omics
One thing we constantly battle in genomics is, “What does it all mean?”
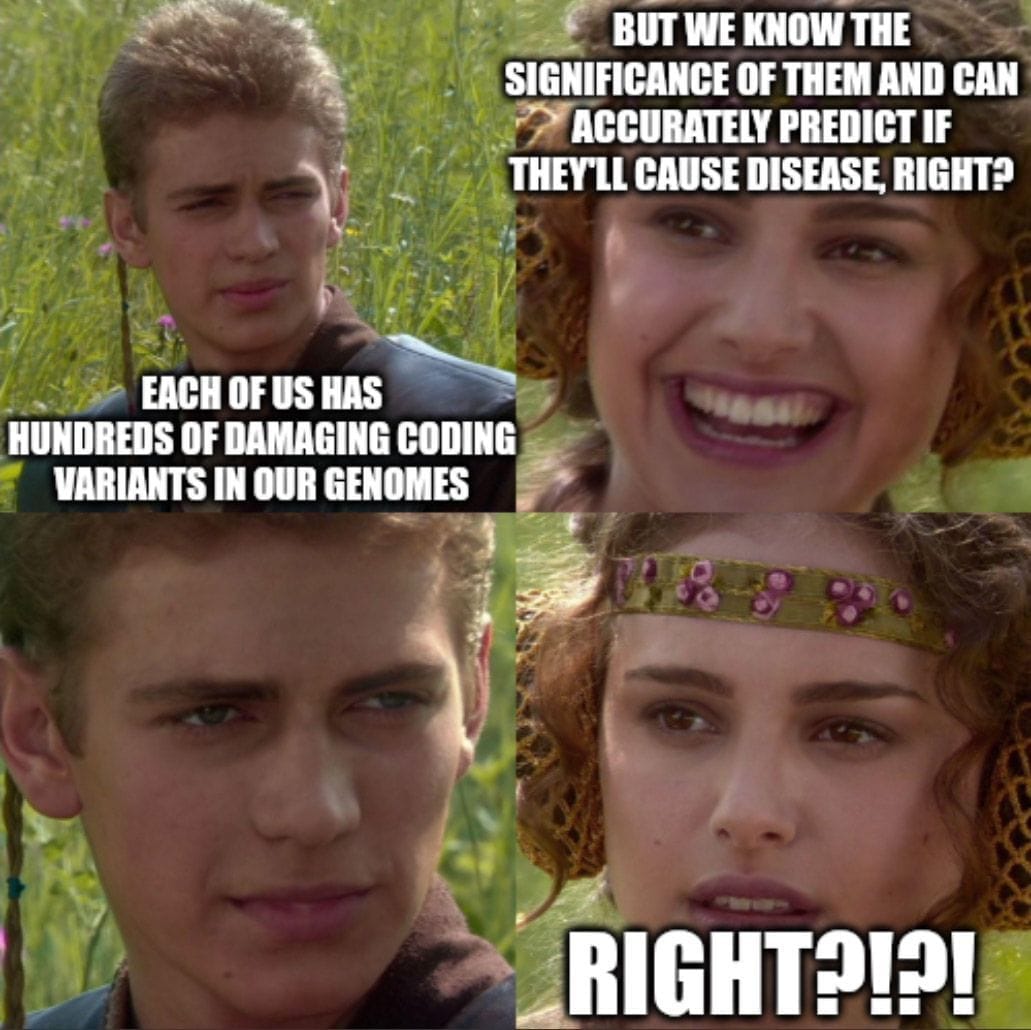
As with everything, it's complicated.
We have 5 million variants in each of our genomes with about 100,000 of those falling in the coding regions of genes.
Of those, a couple hundred are damaging but the majority are what we refer to as variants of unknown significance (VUS).
The process of determining whether those variants cause disease (are pathogenic) or benign (don't cause disease) isn’t incredibly sophisticated.
It’s generally done by looking at families with a history of disease, finding what variants are common in those affected and then deciding with some degree of certainty based on the function of a gene whether or not a variant fits well with the symptoms.
One of the most used databases for assessing whether a variant might cause a disease is ClinVar, and underpinning the sophistication of this process, ClinVar ranks the certainty of a classification with gold stars.
Yes, your kindergarten teacher was onto something after all.
So, it’s probably fair to say we’re still pretty early in the game of figuring out all of this stuff, but too often in genomics we try to find signal in the noise.
Because if there’s a variant, it has to DO something!
And unfortunately, this means we stumble into the pernicious problem of genetic determinism or this idea that the code written in your genome is the only thing that determines whether you will develop a disease.
We realized a while ago that genetics really is only a component here and other genetic, environmental and epigenetic factors all contribute to whether a disease develops (this is called penetrance) and similarly, these factors can also determine the severity of disease in an individual (this is sometimes referred to as expressivity).
However, historically, these measurements were done in families who were affected by a genetic disease so the estimations of penetrance have always been assumed to be over estimates.
Today we can do better because we now have huge population level datasets.
Recent studies have found that about 8% of people carry a pathogenic mutation, but only 7% of those were symptomatic in the EHR.
However, the penetrance of individual mutations varies greatly, for example, on average, 38% of BRCA mutation carriers develop breast cancer.
These results highlight the importance of approaching disease risk associations carefully, especially when reporting results in healthy people.
The genome only ever represents what 'could be,' but observing changes in our other 'omes,' which are the functional product of the genome, can help us better predict when genetic variations might actually manifest as disease.
Because genetics isn't deterministic and for us to truly get a handle on disease and disease progression, we need to marry what we see in the genome with what we can measure in the other 'omes.'