Omic.ly Weekly 14
March 3rd, 2024
Hey There!
Thanks for spending part of your Sunday with Omic.ly!
This week's headlines include:
1) The establishment and maintenance of your microbiome is mostly a family affair, but also, be careful who you swap spit with!
2) Proteomics is a data deluge. Taming the firehose is still a work in progress.
3) RNA interference was a knockout discovery in 1998!
Please enjoy!
What you missed in this week's Premium Edition:
HOT-TAKE: Francis deSouza is back from his post-Illumina purgatory with a new AI start-up
The establishment and maintenance of your microbiome is mostly a family affair, but also, be careful who you swap spit with!
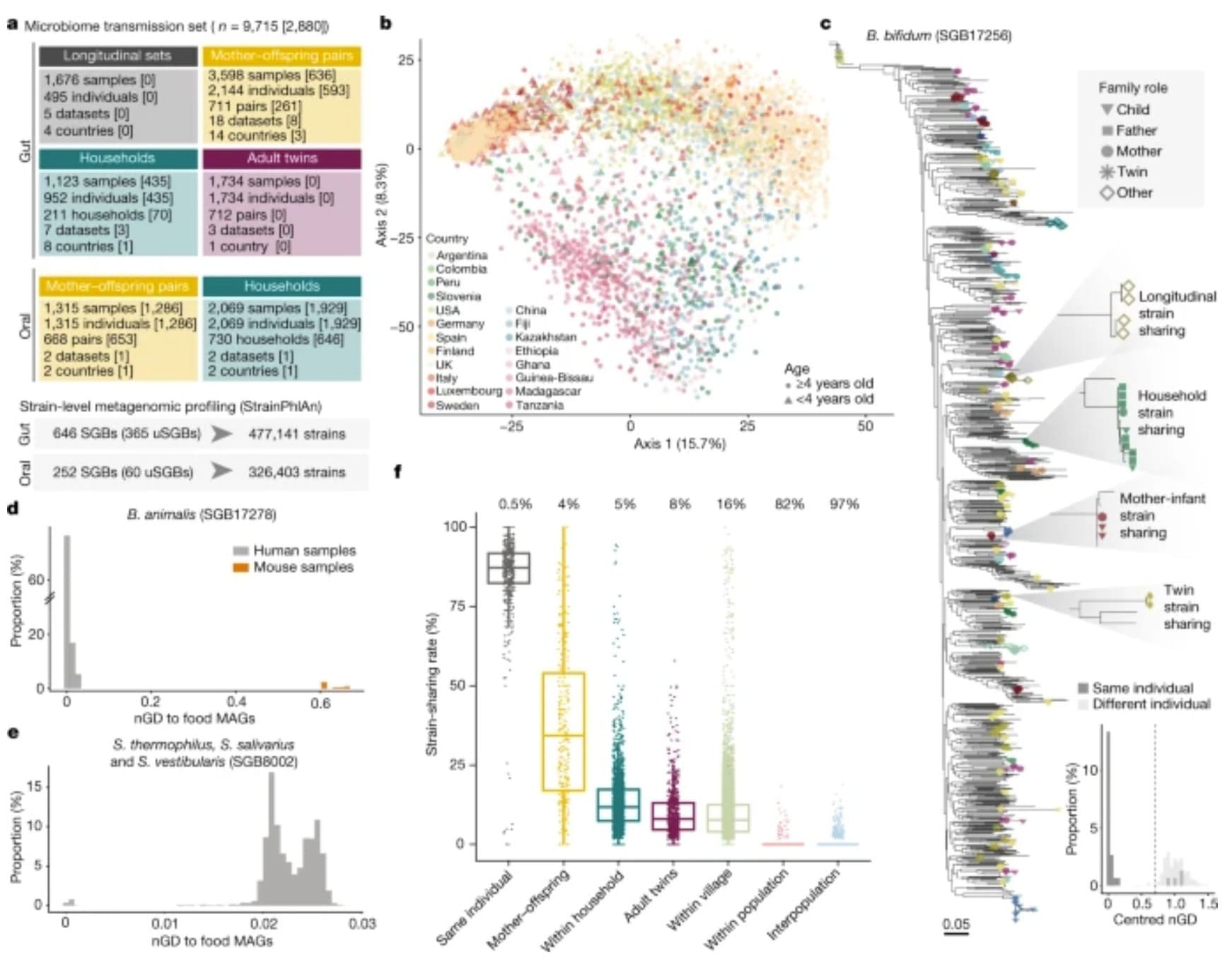
And maybe that’s not super surprising!
Being in close contact with other people is just human nature, so it makes sense that the bugs who colonize us also tend to colonize the people around us.
Our microbiomes and their composition can also be determined by our diets, how much we exercise, or other external environmental and lifestyle factors that alter the unique microenvironments in which they live.
We hear the most about the gut microbiome, but there are many different habitats that bacteria, fungi, and even viruses can occupy in and around our bodies.
Each crevice or orifice has its own!
And to better understand these microbiomes and how they affect human health, we need to understand how they're established.
We know that our first microbiomes are acquired from our moms, but we don’t keep the same ones forever!
And it has been hypothesized that these changes happen through interactions that humans have with other individuals.
However, large scale studies have not been done to explore how microbiome ‘seeding’ occurs at the individual, family, community, and population level.
That’s where today’s paper comes in!
The researchers sought to better understand how our oral and gut microbiomes are established and diversify over time.
The figure below shows a high level overview of their findings. These are summarized in b) where the compositions of microbiomes seem to cluster by geographic region and in f) where microbiomes are least conserved between populations with increasing conservation as you move from the population level to the individuals that live together in the same household.
The researchers found that children share on average 50% of the same microbes as their mother for their first year of life.
This was reduced to 27% at ages 1-3, stabilizing to about 16%.
Interestingly, even adult children who no-longer cohabitate with their mothers still shared about 16% of those microbes, but shared only 8% with other unrelated females!
This is most likely due to family members continuing to share microbiomes with one another because they see each other most often.
And that extends to the other interesting finding that microbiomes were most similar between individuals who lived together (duh…) but that oral microbiomes were best conserved between partners!
Presumably because of all of the kissing.
That should bring a whole new perspective to the shouts of, “EWWWW, GROSS!” the next time your kids catch you and your partner swapping saliva!
###
Valles-Colomer M et al. 2023. The person-to-person transmission landscape of the gut and oral microbiomes. Nature. DOI: 10.1038/s41586-022-05620-1
So, once we have all of this amazing proteomic data, what do we do with it???
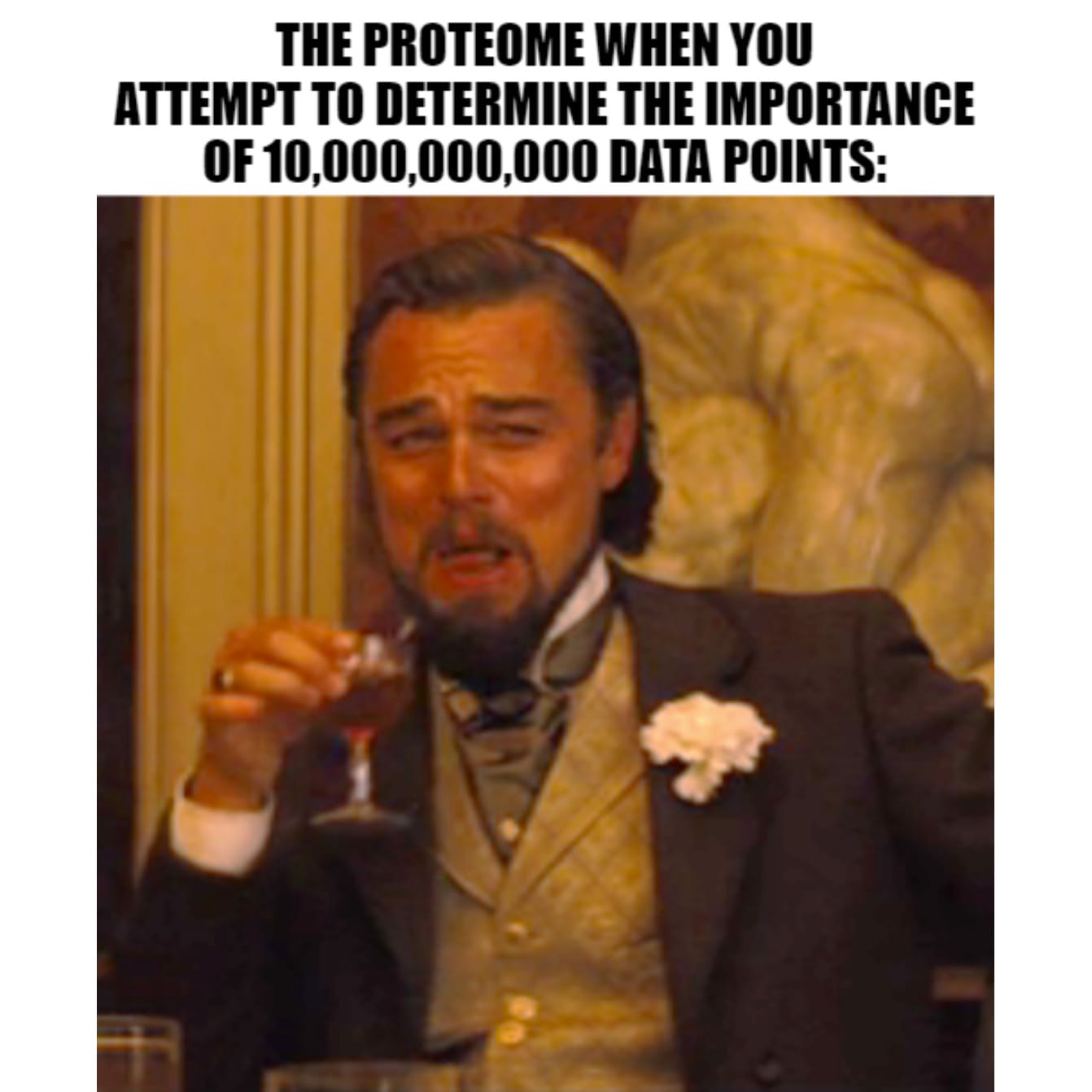
That's a fantastic question!
But your first question might actually be 'What the heck is proteomics!?'
Proteomics is defined as the study of proteins, their functions, regulation and interactions within an organism.
While the genome holds all of our genetic information, the proteome is the genome in action.
And studying the proteome is quite a bit different than studying the genome because the genome is mostly static.
We have no idea what the impact of a mutation or a variant within the genome will be until we see it manifest as a phenotype (a visible symptom, trait or characteristic).
We can see these things on the molecular level by looking at what proteins are produced!
We can figure this out using a variety of techniques including immuno affinity arrays, mass spectrometry and, in the future, protein sequencers.
But once we've gathered the data, what do we do with it and how can we use it to learn anything?
That answer really depends on the experiment that was performed to generate the data. For clinical applications of proteomics I see 3 types of studies being really important:
Longitudinal studies: it's a big word but it just means looking at how things change over time. For example, these could be used to monitor treatment response in oncology patients or detect flares in Crohn's patients.
Case-control studies: these compare diseased individuals to healthy individuals or, diseased tissues to healthy tissues - looking for differences between the two that could be indicative of health or disease.
Single-cell studies: look at how proteins or their interactions change from cell to cell to get a more granular and nuanced view of tissue function, treatment response, or disease presentation.
Analysis is focused on looking at changes over time, among disease states or across tissues.
But a key first step in doing any of these analyses is normalization!
You want to be sure you're comparing apples to apples and that the differences you see aren't just because of some bias that was introduced.
There are a couple of options here, a popular one is to use a protein that is commonly expressed at a static level.
Once everything is normalized you can start digging in!
Differential protein expression: compare how protein levels change from dataset to dataset. These are usually visualized as heat maps.
Pathway analysis: determine what proteins are present, how they're modified, and how they interact with one another. These are visualized as networks or more recently as circos plots.
But one of the biggest drawbacks of doing proteomics is that we're still creating a knowledge base.
Our techniques for looking at the proteome historically have been very low throughput.
Thankfully, that's changing, and new initiatives are helping to provide proteomic references we can use to better hone our analyses!
One of the best ways to figure out what a gene does is to get rid of it and see what happens.
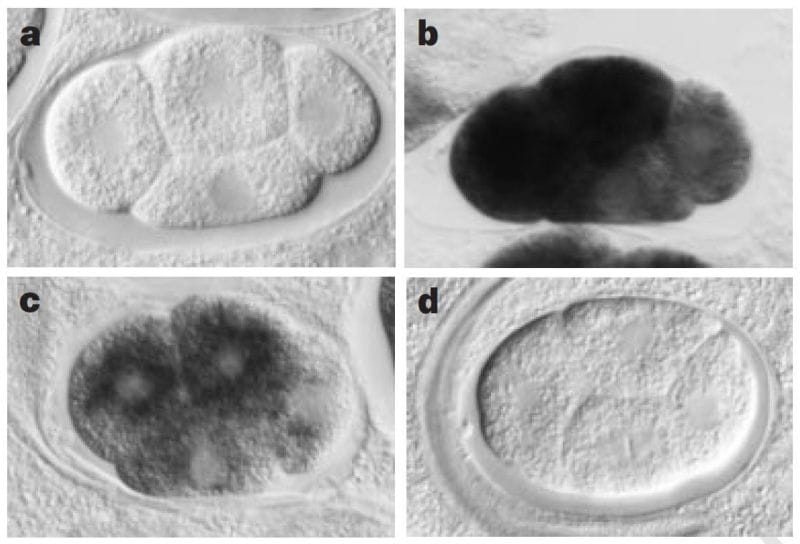
Prior to 1998, this was time consuming and tedious.
That all changed with the discovery of RNA interference.
Gene expression is the process by which the genetic code is converted into something that performs a function within the cell.
Most of the time, this means the conversion of DNA into messenger RNA and then messenger RNA (mRNA) into protein.
It had been known for some time that gene expression could be modified through the introduction of 'anti-sense' or complementary RNA. It was thought that this RNA would bind to the mRNA and prevent it from being read to create protein.
While useful in some biological studies, the effect of anti-sense RNA treatment was modest and the mechanism of how it worked was poorly understood.
So, in 1998, Andy Fire and Craig Mello embarked on a journey to see if they could make this gene expression modification tool more efficient, hypothesizing that the structure of the anti-sense RNA might play a key role.
What they discovered surprised them.
Being good scientists, they introduced anti-sense RNA, sense RNA and both anti-sense and sense together as a double-stranded RNA (dsRNA) complex to determine what effect these combinations would have on gene expression.
The sense and anti-sense treatments had modest effects on their study subject, the nematode worm, C. elegans, but the inclusion of the dsRNA combination had a striking phenotypic effect, mimicking what would have been expected if the genes they were targeting were totally knocked out.
The figure above shows a series of images of a C. elegans embryo. Panel A is the negative control; Panel B shows the staining of an abundant mRNA, mex-3; Panel C shows what happens to mex-3 RNA in the presence of anti-sense RNA; and D shows the complete obliteration of mex-3 RNA after the injection of dsRNA.
Further work after this 1998 paper resulted in the discovery that RNA interference is actually a biological process that cells use to regulate the expression of genes, allowing scientists to identify and name a bunch of new proteins like Drosha, Dicer, Argonaut and the RNA-Induced Silencing Complex (RISC).
We have since co-opted this process for our own uses as a scientific tool and also as a therapeutic for treating human diseases.
Unsurprisingly, given its utility and continued impact, Andy Fire and Craig Mello received the Nobel Prize in Physiology or Medicine in 2006 for their discovery of RNA interference.
###
Fire A et al. 1998. Potent and specific genetic interference by double-stranded RNA in Caenorhabditis elegans. Nature. DOI:10.1038/35888